Citation
Khairudin, Nurkhairizan and Mohd Sharef, Nurfadhlina and Mustapha, Norwati and Mohd Noah, Shahrul Azman
(2018)
Enhancing multi-aspect collaborative filtering for personalized recommendation.
In: 2018 Fourth International Conference on Information Retrieval and Knowledge Management (CAMP'18), 26-28 Mar. 2018, Le Méridien Kota Kinabalu, Sabah, Malaysia. (pp. 91-96).
Abstract
Most existing Collaborative Filtering (CF) approach relies on single overall ratings assigned to items. However, to precisely understand users' behaviours, sometimes this rating alone is not adequate. A user may show his/her overall preferences on an item through the overall ratings but at the same time, they may not satisfy with certain aspects of the item. This situation happened due to the emphasis on aspects may be different among users and will affect a user's final decisions. Therefore, in this paper, we proposed the multi-aspect tensor factorization (MATF) to enhance the predictive accuracy of multi-aspect recommendation by using Tensor Factorization. The evaluation shows that the proposed model outperforms various well-known existing techniques on both single and multi-criteria recommendation.
Download File
![[img]](http://psasir.upm.edu.my/68926/1.hassmallThumbnailVersion/Enhancing%20multi-aspect%20collaborative%20filtering%20for%20personalized%20recommendation.pdf) 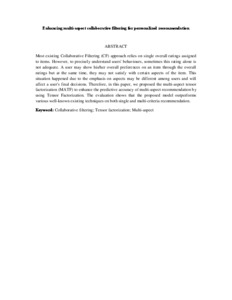 Preview |
|
Text (Abstract)
Enhancing multi-aspect collaborative filtering for personalized recommendation.pdf
Download (35kB)
| Preview
|
|
Additional Metadata
Actions (login required)
 |
View Item |