Citation
Hormozi, Shahram Golzari and C. Doraisamy, Shyamala and Sulaiman, Md. Nasir and Udzir, Nur Izura
(2008)
A hybrid approach to traditional Malay music genre classification: combining feature selection and artificial immune recognition system.
In: 3rd International Symposium on Information Technology (ITSim'08), 26-28 Aug. 2008, Kuala Lumpur, Malaysia. .
Abstract
Music genre classification has a great important role in music information retrieval systems. In this study we propose hybrid approach for Traditional Malay Music (TMM) genre classification. The proposed approach consists of three stages: feature extraction, feature selection and classification with Artificial Immune Recognition System (AIRS). The new version of AIRS is used in this study. In Proposed algorithm, the resource allocation method of AIRS has been changed with a nonlinear method. Based on results of conducted experiments, the obtained classification accuracy of proposed system is 88.6 % using 10 fold cross validation. This accuracy is maximum accuracy among the classifiers used in this study.
Download File
![[img]](http://psasir.upm.edu.my/68896/1.hassmallThumbnailVersion/A%20hybrid%20approach%20to%20traditional%20Malay%20music%20genre%20classification%20combining%20feature%20selection%20and%20artificial%20immune%20recognition%20system.pdf) 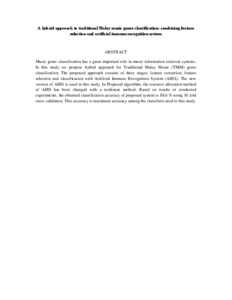 Preview |
|
Text (Abstract)
A hybrid approach to traditional Malay music genre classification combining feature selection and artificial immune recognition system.pdf
Download (34kB)
| Preview
|
|
Additional Metadata
Actions (login required)
 |
View Item |