Citation
Muda, Zaiton and Mohamed Yassin, Warusia and Sulaiman, Md. Nasir and Udzir, Nur Izura
(2011)
Intrusion detection based on K-means clustering and Naïve Bayes classification.
In: 7th International Conference on Information Technology in Asia (CITA 2011), 12-13 July 2011, Kuching, Sarawak. .
Abstract
Intrusion Detection System (IDS) plays an effective way to achieve higher security in detecting malicious activities for a couple of years. Anomaly detection is one of intrusion detection system. Current anomaly detection is often associated with high false alarm with moderate accuracy and detection rates when it's unable to detect all types of attacks correctly. To overcome this problem, we propose an hybrid learning approach through combination of K-Means clustering and Naïve Bayes classification. The proposed approach will be cluster all data into the corresponding group before applying a classifier for classification purpose. An experiment is carried out to evaluate the performance of the proposed approach using KDD Cup'99 dataset. Result show that the proposed approach performed better in term of accuracy, detection rate with reasonable false alarm rate.
Download File
![[img]](http://psasir.upm.edu.my/68866/1.hassmallThumbnailVersion/Intrusion%20detection%20based%20on%20K-means%20clustering%20and%20Na%C3%AFve%20Bayes%20classification.pdf) 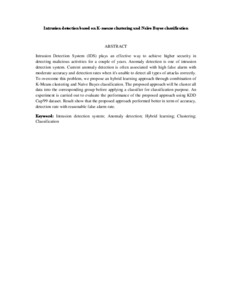 Preview |
|
Text (Abstract)
Intrusion detection based on K-means clustering and Naïve Bayes classification.pdf
Download (35kB)
| Preview
|
|
Additional Metadata
Actions (login required)
 |
View Item |