Citation
Yazdani, Sepideh Foroozan and Azmi Murad, Masrah Azrifah and Mohd Sharef, Nurfadhlina and Singh, Yashwant Prasad and Abdul Latiff, Ahmed Razman
(2017)
Sentiment classification of financial news using statistical features.
International Journal of Pattern Recognition and Artificial Intelligence, 31 (3).
ISSN 0218-0014; ESSN: 1793-6381
Abstract
Sentiment classification of financial news deals with the identification of positive and negative news so that they can be applied in decision support systems for stock trend predictions. This paper explores several types of feature spaces as different data spaces for sentiment classification of the news article. Experiments are conducted using N-gram models unigram, bigram and the combination of unigram and bigram as feature extraction with traditional feature weighting methods (binary, term frequency (TF), and term frequency-document frequency (TF-IDF)), while document frequency (DF) was used in order to generate feature spaces with different dimensions to evaluate N-gram models and traditional feature weighting methods. We performed some experiments to measure the classification accuracy of support vector machine (SVM) with two kernel methods of Linear and Gaussian radial basis function (RBF). We concluded that feature selection and feature weighting methods can have a substantial role in sentiment classification. Furthermore, the results showed that the proposed work which combined unigram and bigram along with TF-IDF feature weighting method and optimized RBF kernel SVM produced high classification accuracy in financial news classification.
Download File
![[img]](http://psasir.upm.edu.my/63198/1.hassmallThumbnailVersion/Sentiment%20classification%20of%20financial%20news%20using%20statistical%20features.pdf) 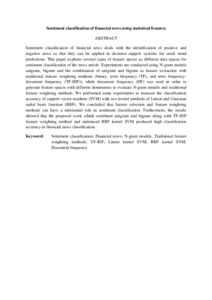 Preview |
|
Text (Abstract)
Sentiment classification of financial news using statistical features.pdf
Download (5kB)
| Preview
|
|
Additional Metadata
Actions (login required)
 |
View Item |