Citation
Yusof, Ahmad Riza'ain and Udzir, Nur Izura and Selamat, Ali and Hamdan, Hazlina and Abdullah @ Selimun, Mohd Taufik
(2017)
Adaptive feature selection for denial of services (DoS) attack.
In: 2017 IEEE Conference on Application, Information and Network Security (AINS), 13-14 Nov. 2017, Miri Marriott Resort & Spa, Miri, Sarawak. (pp. 81-84).
Abstract
Adaptive detection is the learning ability to detect any changes in patterns in intrusion detection systems. In this paper, we propose combining two techniques in feature selection algorithm, namely consistency subset evaluation (CSE) and DDoS characteristic features (DCF) to identify and select the most important and relevant features related DDoS attacks. The proposed technique is trained and tested using the NSL-KDD 2009 dataset and compared with the traditional features selection method such as Information Gain, Gain Ratio, Chi-squared and Correlated features selection (CFS). The result shows that the combined CSE with DCF model overcomes the drawback of traditional feature selection technique such as avoid over-fitting, long training time and improved efficiency of detections. The adaptive model based on this technique can reduce computational complexity to analyze the data when attack occurs.
Download File
![[img]](http://psasir.upm.edu.my/59480/1.hassmallThumbnailVersion/Adaptive%20feature%20selection%20for%20denial%20of%20services%20%28DoS%29%20attack.pdf) 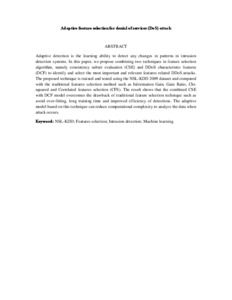 Preview |
|
Text (Abstract)
Adaptive feature selection for denial of services (DoS) attack.pdf
Download (34kB)
| Preview
|
|
Additional Metadata
Actions (login required)
 |
View Item |