Citation
Srazhidinov, Radik and Raja Ahmad, Raja Mohd Kamil
(2016)
Performance comparison of THF-NLFXLMS and VFXLMS algorithms for Hammerstein NANC.
In: 2016 IEEE Conference on Systems, Process and Control (ICSPC 2016), 16-18 Dec. 2016, Melaka, Malaysia. (pp. 76-79).
Abstract
Recently, THF-NLFXLMS algorithm was developed to compensate the nonlinearity encountered in nonlinear active noise control systems. Despite similar performance, this algorithm is more advantageous than the Nonlinear Filtered-X Least Mean Square (NLFXLMS) due to the use of tangential hyperbolic function (THF) instead of scaled error function (SEF) which allows the degree of nonlinearity to be modeled. In addition, the computational complexity is relatively small compared to other direct nonlinear adaptive algorithm like the Volterra filter. In this paper, the performance of THF-NLFXLMS algorithm for Hammerstein secondary path structure is quantified and compared with NLFXLMS and the Volterra Filtered-x Least Mean Squares (VFXLMS) algorithm of similar computational complexity. The results show that the THF-NLFXLMS algorithm has similar performance as NLFXLMS algorithm and outperforms 2nd order VFXLMS algorithm.
Download File
![[img]](http://psasir.upm.edu.my/56107/1.hassmallThumbnailVersion/Performance%20comparison%20of%20THF-NLFXLMS%20and%20VFXLMS%20algorithms%20for%20Hammerstein%20NANC.pdf) 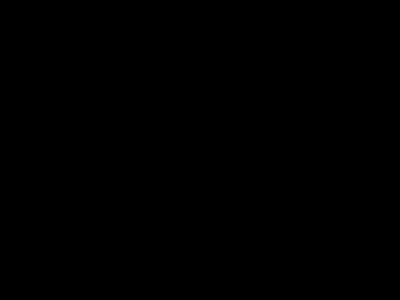 Preview |
|
PDF (Abstract)
Performance comparison of THF-NLFXLMS and VFXLMS algorithms for Hammerstein NANC.pdf
Download (37kB)
| Preview
|
|
Additional Metadata
Actions (login required)
 |
View Item |