Citation
Mohd Pozi, Muhammad Syafiq and Sulaiman, Md. Nasir and Mustapha, Norwati and Perumal, Thinagaran
(2016)
Improving anomalous rare attack detection rate for intrusion detection system using support vector machine and genetic programming.
Neural Processing Letters, 44 (2).
pp. 279-290.
ISSN 1370-4621; ESSN: 1573-773X
Abstract
Commonly addressed problem in intrusion detection system (IDS) research works that employed NSL-KDD dataset is to improve the rare attacks detection rate. However, some of the rare attacks are hard to be recognized by the IDS model due to their patterns are totally missing from the training set, hence, reducing the rare attacks detection rate. This problem of missing rare attacks can be defined as anomalous rare attacks and hardly been solved in IDS literature. Hence, in this letter, we proposed a new classifier to improve the anomalous attacks detection rate based on support vector machine (SVM) and genetic programming (GP). Based on the experimental results, our classifier, GPSVM, managed to get higher detection rate on the anomalous rare attacks, without significant reduction on the overall accuracy. This is because, GPSVM optimization task is to ensure the accuracy is balanced between classes without reducing the generalization property of SVM.
Download File
![[img]](http://psasir.upm.edu.my/54525/1.hassmallThumbnailVersion/Improving%20anomalous%20rare%20attack%20detection%20rate%20for%20intrusion%20detection%20system%20using%20support%20vector%20machine%20and%20genetic%20programming.pdf) 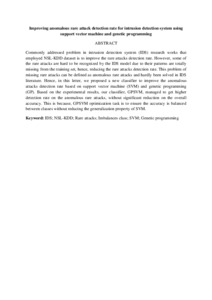 Preview |
|
Text
Improving anomalous rare attack detection rate for intrusion detection system using support vector machine and genetic programming.pdf
Download (5kB)
| Preview
|
|
Additional Metadata
Actions (login required)
 |
View Item |