Citation
Zand, Mohsen and Doraisamy, Shyamala and Abdul Halin, Alfian and Mustaffa, Mas Rina
(2016)
Ontology-based semantic image segmentation using mixture models and multiple CRFs.
IEEE Transactions on Image Processing, 25 (7).
pp. 3233-3248.
ISSN 1057-7149, ESSN: 1941-0042
Abstract
Semantic image segmentation is a fundamental yet challenging problem, which can be viewed as an extension of the conventional object detection with close relation to image segmentation and classification. It aims to partition images into non-overlapping regions that are assigned predefined semantic labels. Most of the existing approaches utilize and integrate low-level local features and high-level contextual cues, which are fed into an inference framework such as, the conditional random field (CRF). However, the lack of meaning in the primitives (i.e., pixels or superpixels) and the cues provides low discriminatory capabilities, since they are rarely object-consistent. Moreover, blind combinations of heterogeneous features and contextual cues exploitation through limited neighborhood relations in the CRFs tend to degrade the labeling performance. This paper proposes an ontology-based semantic image segmentation (OBSIS) approach that jointly models image segmentation and object detection. In particular, a Dirichlet process mixture model transforms the low-level visual space into an intermediate semantic space, which drastically reduces the feature dimensionality. These features are then individually weighed and independently learned within the context, using multiple CRFs. The segmentation of images into object parts is hence reduced to a classification task, where object inference is passed to an ontology model. This model resembles the way by which humans understand the images through the combination of different cues, context models, and rule-based learning of the ontologies. Experimental evaluations using the MSRC-21 and PASCAL VOC'2010 data sets show promising results.
Download File
![[img]](http://psasir.upm.edu.my/53436/1.hassmallThumbnailVersion/Ontology-based%20semantic%20image%20segmentation%20using%20mixture%20models%20and%20multiple%20CRFs.pdf) 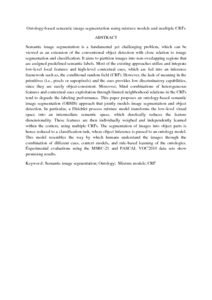 Preview |
|
PDF
Ontology-based semantic image segmentation using mixture models and multiple CRFs.pdf
Download (6kB)
| Preview
|
|
Additional Metadata
Actions (login required)
 |
View Item |