Citation
Haoyuan, Hong and Pradhan, Biswajeet and Chong, Xu and Dieu, Tien Bui
(2015)
Spatial prediction of landslide hazard at the Yihuang area (China) using two-class kernel logistic regression, alternating decision tree and support vector machines.
Catena, 133.
pp. 266-281.
ISSN 0341-8162; ESSN: 1872-6887
Abstract
Preparation of landslide susceptibility map is the first step for landslide hazard mitigation and risk assessment.
The main aim of this study is to explore potential applications of two new models such as two-class Kernel
Logistic Regression (KLR) and Alternating Decision Tree (ADT) for landslide susceptibility mapping at the
Yihuang area (China). The ADT has not been used in landslide susceptibility modeling and this paper attempts
a novel application of this technique. For the purpose of comparison, a conventional method of Support Vector
Machines (SVM) which has been widely used in the literature was included and their results were assessed. At first, a landslide inventory map with 187 landslide locations for the study area was constructed from various sources. Landslide locations were then spatially randomly split in a ratio of 70/30 for building landslide models and for the model validation. Then a spatial database with a total of fourteen landslide conditioning factors was prepared, including slope, aspect, altitude, topographic wetness index (TWI), stream power index (SPI),sediment transport index (STI), plan curvature, land use, normalized difference vegetation index (NDVI), lithology, distance to faults, distance to rivers, distance to roads, and rainfall. Using the KLR, the SVM, and the ADT, three landslide susceptibility models were constructed using the training dataset. The three resulting models were validated and compared using the receive operating characteristic (ROC), Kappa index, and five statistical evaluation measures. In addition, pairwise comparisons of the area under the ROC curve were carried out to assess if there are significant differences on the overall performance of the three models. The goodness-of-
fits are 92.5%(the KLR model), 88.8% (the SVM model), and 95.7% (the ADT model). The prediction capabilities are 81.1%,84.2%, and 93.3% for the KLR, the SVM, and the ADT models, respectively. The result shows that the ADT model
yielded better overall performance and accurate results than the KLR and SVM models. The KLR model considered
slightly better than SVM model in terms of the positive prediction values. The ADT and KLR are the two promising
data mining techniques which might be considered to use in landslide susceptibility mapping. The results from
this study may be useful for land use planning and decision making in landslide prone areas.
Download File
![[img]](http://psasir.upm.edu.my/46353/1.hassmallThumbnailVersion/Spatial%20prediction%20of%20landslide%20hazard%20at%20the%20Yihuang%20area%20%28China%29%20using%20two-class%20kernel%20logistic%20regression%2C%20alternating%20decision%20tree%20and%20support%20vector%20machines.pdf) 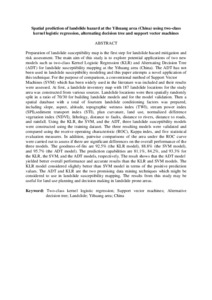 Preview |
|
Text (Abstract)
Spatial prediction of landslide hazard at the Yihuang area (China) using two-class kernel logistic regression, alternating decision tree and support vector machines.pdf
Download (7kB)
| Preview
|
|
Additional Metadata
Actions (login required)
 |
View Item |