Citation
Zulkifli, Noor Aishah Atiqah and Md. Ali, Sawal Hamid and Ahmad, Siti Anom and Islam, Md Shabiul
(2015)
Review on support vector machine (SVM) classifier for human emotion pattern recognition from EEG signals.
Asian Journal of Information Technology, 14 (4).
pp. 135-146.
ISSN 1682-3915; ESSN: 1993-5994
Abstract
This study reviewed the strategy in pattern classification for human emotion recognition system based on Support Vector Machine (SVM) classifier on Electroencephalography (EEG) signal. SVM has been widely used as a classifier and has been reported as having minimum error and produce accurate classification. However, the accuracy is influenced by many factors such as the electrode placement, equipment used, preprocessing techniques and selection of feature extraction methods. There are many types of SVM classifier such as SVM via Radial Basis Function (RBF), Linear Support Vector Machine (LSVM) and Multiclass Least Squares Support Vector Machine (MC-LS-SVM). SVM via RBF states the average accuracy rate of 92.73, 85.41, 93.80 and 67.40% using different features extraction method, respectively. The accuracy using LSVM and MC-LS-SVM classifier are 91.04 and 77.15%, respectively. Although, the accuracy rate influenced by many factors in the experimental works, SVM always shows their function as a great classifier. This study will discuss and summarize a few related works of EEG signals in classifying human emotion using SVM classifier.
Download File
![[img]](http://psasir.upm.edu.my/46176/1.hassmallThumbnailVersion/Review%20on%20support%20vector%20machine%20%28SVM%29%20classifier%20for%20human%20emotion%20pattern%20recognition%20from%20EEG%20signals.pdf) 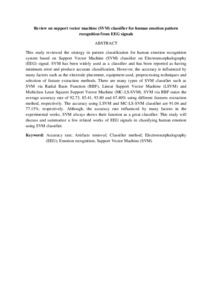 Preview |
|
Text (Abstract)
Review on support vector machine (SVM) classifier for human emotion pattern recognition from EEG signals.pdf
Download (5kB)
| Preview
|
|
Additional Metadata
Actions (login required)
 |
View Item |