Citation
Khandanlou, Roshanak and Masoumi, Hamid Reza Fard and Ahmad @ Ayob, Mansor and Shameli, Kamyar and Basri, Mahiran and Kalantari, Katayoon
(2016)
Enhancement of heavy metals sorption via nanocomposites of rice straw and Fe3O4 nanoparticles using artificial neural network (ANN).
Ecological Engineering, 91.
pp. 249-256.
ISSN 0925-8574; ESSN: 1872-6992
Abstract
The artificial neural network (ANN) modeling of adsorption of Pb(II) and Cu(II) was carried out for determination of the optimum values of the variables to get the maximum removal efficiency. The input variables were initial ion concentration, adsorbent dosage, and removal time, while the removal efficiency was considered as output. The performed experiments were designed into two data sets including training, and testing sets. To acquire the optimum topologies, ANN was trained by quick propagation (QP), Batch Back Propagation (BBP), Incremental Back Propagation (IBP), genetic algorithm (GA) and Levenberg-Marquardt (LM) algorithms for testing data set. The topologies were defined by the indicator of minimized root mean squared error (RMSE) for each algorithm. According to the indicator, the IBP-3-9-2 was selected as the optimized topologies for heavy metal removal, due to the minimum RMSE and maximum R-squared.
Download File
![[img]](http://psasir.upm.edu.my/43223/1.hassmallThumbnailVersion/Enhancement%20of%20heavy%20metals%20sorption%20via%20nanocomposites%20of%20rice%20straw%20and%20Fe3O4%20nanoparticles%20using%20artificial%20neural%20network%20%28ANN%29.pdf) 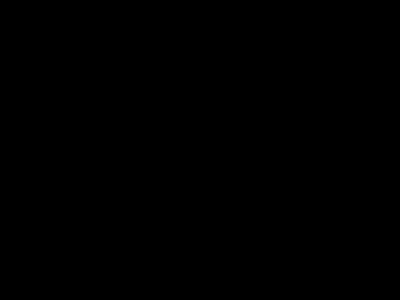 Preview |
|
PDF (Abstract)
Enhancement of heavy metals sorption via nanocomposites of rice straw and Fe3O4 nanoparticles using artificial neural network (ANN).pdf
Download (37kB)
| Preview
|
|
Additional Metadata
Actions (login required)
 |
View Item |