Citation
Mohd Amin, Nor Azrita and Adam, Mohd Bakri and Ibrahim, Noor Akma
(2013)
Prior selection for Gumbel distribution parameters using multiple-try metropolis algorithm for monthly maxima PM10 data.
In: Statistics and Operational Research International Conference (SORIC 2013), 3–5 Dec. 2013, Sarawak, Malaysia. (pp. 317-324).
Abstract
The Multiple-try Metropolis (MTM) algorithm is the new alternatives in the field of Bayesian extremes for summarizing the posterior distribution. MTM produce efficient estimation scheme for modelling extreme data in term of the convergence and small burn-in periods. The main objective is to explore the accuracy of the parameter estimation to the change of priors and compare the results with a classical likelihood-based analysis. Focus is on modelling the extreme data based on block maxima approach using Gumbel distribution. The comparative study between MTM and MLE is shown by the numerical problems. Several goodness of fit tests are compute for selecting the best model. The application is on the monthly maxima PM10 data for Johor state.
Download File
![[img]](http://psasir.upm.edu.my/35055/1.hassmallThumbnailVersion/Prior%20selection%20for%20Gumbel%20distribution%20parameters%20using%20multiple-try%20metropolis%20algorithm%20for%20monthly%20maxima%20PM10%20data.pdf) 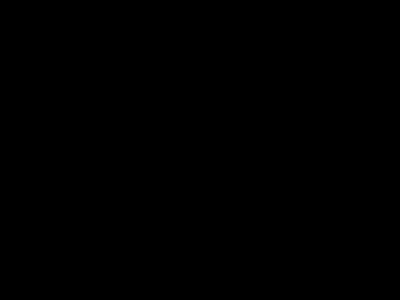 Preview |
|
PDF (Abstract)
Prior selection for Gumbel distribution parameters using multiple-try metropolis algorithm for monthly maxima PM10 data.pdf
Download (36kB)
| Preview
|
|
Additional Metadata
Actions (login required)
 |
View Item |