Citation
Jaafar, Mohd Saleh and Noorzaei, Jamaloddin and Jameel, Mohammed and Seyed Hakim, Seyed Jamalaldin and Mohammadhassani, Mohammad
(2011)
Application of artificial neural networks to predict compressive strength of high strength concrete.
International Journal of Physical Sciences, 6 (5).
art. no. 64E466B26591 .
pp. 975-981.
ISSN 1992-1950
Abstract
A method to predict 28-day compressive strength of high strength concrete (HSC) by using MFNNs is proposed in this paper. The artificial neural networks (ANN) model is constructed trained and tested using the available data. A total of 368 different data of HSC mix-designs were collected from technical literature. The data used to predict the compressive strength with ANN consisted of eight input parameters which include cement, water, coarse aggregate, fine aggregate, silica fume, superplasticizer, fly ash and granulated grated blast furnace slag. For the training phase, different combinations of layers, number of neurons, learning rate, momentum and activation functions were considered. The training was terminated when the root mean square error (RMSE) reached or was less than 0.001 and the results were tested with test data set. A total of 30 architectures were studied and the 8-10-6-1 architecture was the best possible architecture. The results show that the relative percentage error (RPE) for the training set was 7.02% and the testing set was 12.64%.The ANNs models give high prediction accuracy, and the research results demonstrate that using ANNs to predict concrete strength is practical and beneficial.
Download File
![[img]](http://psasir.upm.edu.my/23423/1.hassmallThumbnailVersion/Application%20of%20artificial%20neural%20networks%20to%20predict%20compressive%20strength%20of%20high%20strength%20concrete.pdf) 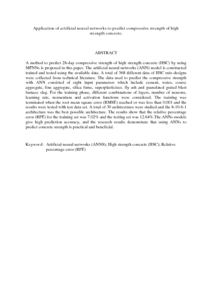 Preview |
|
PDF (Abstract)
Application of artificial neural networks to predict compressive strength of high strength concrete.pdf
Download (85kB)
| Preview
|
|
Additional Metadata
Actions (login required)
 |
View Item |