Citation
Mustapha, Norwati and Jalali, Manijeh and Jalali, Mehrdad
(2009)
Expectation maximization clustering algorithm for user modeling in web usage mining system.
European Journal of Scientific Research, 32 (4).
pp. 467-476.
ISSN 1450-216X
Abstract
To provide intelligent personalized online services such as web recommender systems, it is usually necessary to model users’ web access behavior. To achieve this, one of the promising approaches is web usage mining, which mines web logs for user models and recommendations. Web usage mining algorithms have been widely utilized for modeling user web navigation behavior. In this study we advance a model for mining of user’s navigation pattern. The model is based on expectation-maximization (EM) algorithm and it is used for finding maximum likelihood estimates of parameters in probabilistic models, where the model depends on unobserved latent variables. The experimental results represent that by decreasing the number of clusters, the log likelihood converges toward lower values and probability of the largest cluster will be decreased while the number of
the clusters increases in each treatment. The results also indicate that kind of behavior given by EM clustering algorithm has improved the visit-coherence (accuracy) of
navigation pattern mining.
Download File
![[img]](http://psasir.upm.edu.my/14638/1.hassmallThumbnailVersion/Expectation%20maximization%20clustering%20algorithm%20for%20user%20modeling%20in%20web%20usage%20mining%20system.pdf) 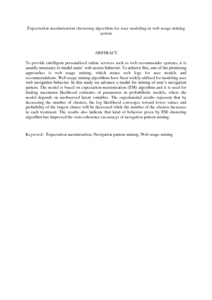 Preview |
|
PDF (Abstract)
Expectation maximization clustering algorithm for user modeling in web usage mining system.pdf
Download (177kB)
| Preview
|
|
Additional Metadata
Actions (login required)
 |
View Item |