Citation
Yazdani, Sepideh Foroozan and Azmi Murad, Masrah Azrifah and Mohd Sharef, Nurfadhlina and Abdul Latiff, Ahmed Razman
(2015)
Improving sentiment classification accuracy of financial news using n-gram approach and feature weighting methods.
In: 2nd International Conference on Information Science and Security (ICISS 2015), 14-16 Dec. 2015, Seoul, South Korea. .
Abstract
Sentiment classification of financial news deals with the identification of positive and negative news so that they can be applied in decision support system to perform stock trend predictions. This paper explores several types of feature space as different datasets for sentiment classification of the news article. Experiments are conducted based on n-gram approach (unigram, bigram and the combination of unigram and bigram) used as feature extraction with different feature weighting methods, while, document frequency (DF) is used as feature selection method. We performed experiments to measure the classification accuracy of support vector machine (SVM) with two kernel methods of linear and Radial Basis Function (RBF). Results showed that an efficient feature extraction increased classification accuracy when it is used as a combination of unigram and bigram. Moreover, we also found that DF can be applied as a dimension reduction method to reduce the feature space without loss of accuracy.
Download File
![[img]](http://psasir.upm.edu.my/14342/1.hassmallThumbnailVersion/Improving%20sentiment%20classification%20accuracy%20of%20financial%20news%20using%20n-gram%20approach%20and%20feature%20weighting%20methods.pdf) 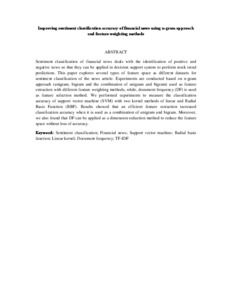 Preview |
|
Text (Abstract)
Improving sentiment classification accuracy of financial news using n-gram approach and feature weighting methods.pdf
Download (35kB)
| Preview
|
|
Additional Metadata
Actions (login required)
 |
View Item |