Citation
Salari, Nader and Shohaimi, Shamarina and Najafi, Farid and Nallappan, Meenakshii and Karishnarajah, Isthrinayagy
(2012)
An improved artificial neural network based model for prediction of late onset heart failure.
Life Science Journal, 9 (4).
pp. 3684-3689.
ISSN 1097-8135; ESSN: 2372-613X
Abstract
Background and Objective: The present study aims to present an artificial neural network (ANN)-based model for prediction of Late Onset Heart Failure (LOHF) in patients, with no previous Heart Failure (HF) history, who experienced non-fatal, first-ever Acute Myocardial Infarction (AMI) without previous history of heart failure. Methods: Two models of multilayer perceptron (MLP) and Radial Basis Function (RBF) neural network approaches based on decision support system were developed. The MLP model was used to optimize the predicting algorithm based on the conjugate gradients descent method. To design the RBF network, K-Means clustering technique was used to select the centers of RBFs, and k-nearest neighbourhood to define the spread and forward selection for determining the optimum number of RBFs. To assess the generalization of the network, K-fold cross-validation test was used. A total of 3,109 medical records containing 19 main clinical parameters were used to train and test the networks. Results: The findings indicate a reliable performance of the proposed system. The MLP based model yields a sensitivity, specificity, and an area under the receiver/relative operating characteristic (ROC) curve (AUC) of 87.1%, 90%, and 0.887 ± 0.02, respectively. However, the RBF network shows the above parameters as 84.4%, 94.3%, and 0.905 ± 0.017, respectively. Conclusions: The proposed intelligence system achieved a high degree of diagnostic accuracy (92.9% for MLP and 93.7% for RBF) indicating its high efficiency for clinical diagnosis of LOHF.
Download File
![[img]](http://psasir.upm.edu.my/13846/1.hassmallThumbnailVersion/An%20improved%20artificial%20neural%20network%20based%20model%20for%20prediction%20of%20late%20onset%20heart%20failure.pdf) 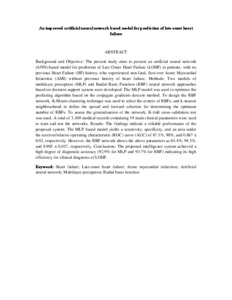 Preview |
|
Text (Abstract)
An improved artificial neural network based model for prediction of late onset heart failure.pdf
Download (50kB)
| Preview
|
|
Additional Metadata
Actions (login required)
 |
View Item |