Citation
Muda, Zaiton and Yassin, Warusia and Sulaiman, Md. Nasir and Udzir, Nur Izura
(2011)
A K-Means and Naive Bayes learning approach for better intrusion detection.
Information Technology Journal, 10 (3).
pp. 648-655.
ISSN 1812-5638; ESSN: 1812-5646
Abstract
Intrusion Detection Systems (IDS) have become an important building block of any sound defense network infrastructure. Malicious attacks have brought more adverse impacts on the networks than before, increasing the need for an effective approach to detect and identify such attacks more effectively. In this study two learning approaches, K-Means Clustering and Naïve Bayes classifier (KMNB) are used to perform intrusion detection. K-Means is used to identify groups of samples that behave similarly and dissimilarly such as malicious and non-malicious activity in the first stage while Naive Bayes is used in the second stage to classify all data into correct class category. Experiments were performed with KDD Cup '99 data sets. The experimental results show that KMNB significantly improved and increased the accuracy, detection rate and false alarm of single Naïve Bayes classifier up to 99.6, 99.8 and 0.5%.
Download File
![[img]](http://psasir.upm.edu.my/12710/1.hassmallThumbnailVersion/A%20K-Means%20and%20Naive%20Bayes%20learning%20approach%20for%20better%20intrusion%20detection.pdf) 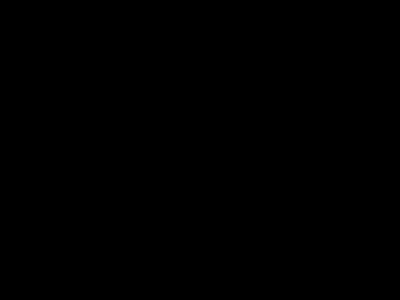 Preview |
|
PDF (Abstract)
A K-Means and Naive Bayes learning approach for better intrusion detection.pdf
Download (36kB)
| Preview
|
|
Additional Metadata
Actions (login required)
 |
View Item |