Citation
N. A., Lili
(2009)
A framework for human action detection via extraction of multimodal features.
International Journal of Image Processing, 3 (2).
pp. 73-79.
ISSN 1985-2304
Abstract
This work discusses the application of an Artificial Intelligence technique called data extraction and a process-based ontology in constructing experimental
qualitative models for video retrieval and detection. We present a framework architecture that uses multimodality features as the knowledge representation scheme to model the behaviors of a number of human actions in the video
scenes. The main focus of this paper placed on the design of two main components (model classifier and inference engine) for a tool abbreviated as VASD (Video Action Scene Detector) for retrieving and detecting human actions
from video scenes. The discussion starts by presenting the workflow of the retrieving and detection process and the automated model classifier construction logic. We then move on to demonstrate how the constructed classifiers can be
used with multimodality features for detecting human actions. Finally, behavioral explanation manifestation is discussed. The simulator is implemented in bilingual;
Math Lab and C++ are at the backend supplying data and theories while Java handles all front-end GUI and action pattern updating. To compare the usefulness of the proposed framework, several experiments were conducted and
the results were obtained by using visual features only (77.89% for precision; 72.10% for recall), audio features only (62.52% for precision; 48.93% for recall) and combined audiovisual (90.35% for precision; 90.65% for recall).
Download File
![[img]](http://psasir.upm.edu.my/12694/1.hassmallThumbnailVersion/A%20framework%20for%20human%20action%20detection%20via%20extraction%20of%20multimodal%20features.pdf) 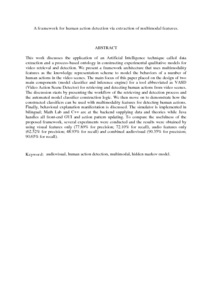 Preview |
|
PDF (Abstract)
A framework for human action detection via extraction of multimodal features.pdf
Download (84kB)
| Preview
|
|
Additional Metadata
Actions (login required)
 |
View Item |