Citation
Omar, Muhammad Azhar and Hassan, Mohd Khair and Che Soh, Azura and Ab Kadir, Mohd Zainal Abidin
(2013)
Lightning severity classification utilizing the meteorological parameters: a neural network approach.
In: 2013 IEEE International Conference on Control System, Computing and Engineering (ICCSCE 2013), 29 Nov.-1 Dec. 2013, Penang, Malaysia. (pp. 111-116).
Abstract
This paper presents a technique of predicting lightning severity on daily basis by using meteorological data. The data used is supplied by Global Lightning Network (GLN) from WSI Corporation. The input of the system consists of seven meteorology parameters which had been provided by Malaysia Meteorology Service with minimal fees. Input parameters are the Minimum Humidity, Maximum Humidity, Minimum Temperature, Maximum Temperature, Rainfall, Week and Month. The output of the system determines the severity of lightning predictions in three stages; Class1: Hazardous; Class2: Warning; and Class3: Low Risk. Two training algorithms that have been tested in this study namely the Gradient Descent with Momentum Backpropagation (traingdm) and the Scaled Conjugated Gradient Backpropagation (trainscg). The traingdm has indicated better accuracy of 70% compared to the trainscg whilst in contrast; trainscg has demonstrated approximately 4 times faster training compare to traingdm.
Download File
![[img]](http://psasir.upm.edu.my/68852/1.hassmallThumbnailVersion/Lightning%20severity%20classification%20utilizing%20the%20meteorological%20parameters%20a%20neural%20network%20approach.pdf) 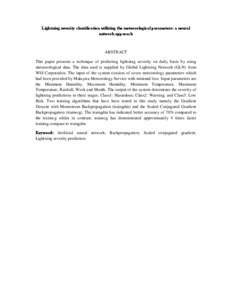 Preview |
|
Text (Abstract)
Lightning severity classification utilizing the meteorological parameters a neural network approach.pdf
Download (36kB)
| Preview
|
|
Additional Metadata
Actions (login required)
 |
View Item |