Citation
Yassin, Warusia and Udzir, Nur Izura and Abdullah, Azizol and Abdullah @ Selimun, Mohd Taufik and Zulzalil, Hazura and Muda, Zaiton
(2014)
Signature-based anomaly intrusion detection using integrated data mining classifiers.
In: International Symposium on Biometrics and Security Technologies (ISBAST 2014), 26-27 Aug. 2014, Kuala Lumpur, Malaysia. (pp. 232-237).
Abstract
As the influence of Internet and networking technologies as communication medium advance and expand across the globe, cyber attacks also grow accordingly. Anomaly detection systems (ADSs) are employed to scrutinize information such as packet behaviours coming from various locations on network to find those intrusive activities as fast as possible with precision. Unfortunately, besides minimizing false alarms; the performance issues related to heavy computational process has become drawbacks to be resolved in this kind of detection systems. In this work, a novel Signature-Based Anomaly Detection Scheme (SADS) which could be applied to scrutinize packet headers' behaviour patterns more precisely and promptly is proposed. Integrating data mining classifiers such as Naive Bayes and Random Forest can be utilized to decrease false alarms as well as generate signatures based on detection results for future prediction and reducing processing time. Results from a number of experiments using DARPA 1999 and ISCX 2012 benchmark dataset have validated that SADS own better detection capabilities with lower processing duration as contrast to conventional anomaly-based detection method.
Download File
![[img]](http://psasir.upm.edu.my/47759/1.hassmallThumbnailVersion/Signature-based%20anomaly%20intrusion%20detection%20using%20integrated%20data%20mining%20classifiers.pdf) 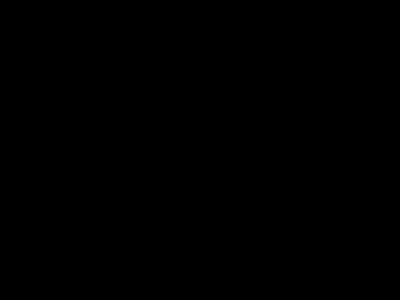 Preview |
|
PDF (Abstract)
Signature-based anomaly intrusion detection using integrated data mining classifiers.pdf
Download (34kB)
| Preview
|
|
Additional Metadata
Actions (login required)
 |
View Item |