Citation
Kakavand, Mohsen and Mustapha, Norwati and Mustapha, Aida and Abdullah @ Selimun, Mohd Taufik
(2016)
Effective dimensionality reduction of payload-based anomaly detection in TMAD model for HTTP payload.
KSII Transactions on Internet and Information Systems, 10 (8).
pp. 1-27.
ISSN 1976-7277
Abstract
Intrusion Detection System (IDS) in general considers a big amount of data that are highly redundant and irrelevant. This trait causes slow instruction, assessment procedures, high resource consumption and poor detection rate. Due to their expensive computational requirements during both training and detection, IDSs are mostly ineffective for real-time anomaly detection. This paper proposes a dimensionality reduction technique that is able to enhance the performance of IDSs up to constant time O(1) based on the Principle Component Analysis (PCA). Furthermore, the present study offers a feature selection approach for identifying major components in real time. The PCA algorithm transforms high-dimensional feature vectors into a low-dimensional feature space, which is used to determine the optimum volume of factors. The proposed approach was assessed using HTTP packet payload of ISCX 2012 IDS and DARPA 1999 dataset. The experimental outcome demonstrated that our proposed anomaly detection achieved promising results with 97% detection rate with 1.2% false positive rate for ISCX 2012 dataset and 100% detection rate with 0.06% false positive rate for DARPA 1999 dataset. Our proposed anomaly detection also achieved comparable performance in terms of computational complexity when compared to three state-of-the-art anomaly detection systems.
Download File
![[img]](http://psasir.upm.edu.my/54067/1.hassmallThumbnailVersion/Effective%20dimensionality%20reduction%20of%20payload-based%20anomaly%20detection%20in%20TMAD%20model%20for%20HTTP%20payload.pdf) 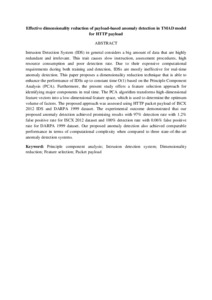 Preview |
|
Text
Effective dimensionality reduction of payload-based anomaly detection in TMAD model for HTTP payload.pdf
Download (7kB)
| Preview
|
|
Additional Metadata
Actions (login required)
 |
View Item |