Citation
Iqbal, Rizwan and Azmi Murad, Masrah Azrifah and Mustapha, Aida and Panahy, Payam Hassany Shariat and Khanahmadliravi, Nasim
(2013)
An experimental study of classification algorithms for crime prediction.
Indian Journal of Science and Technology, 6 (3).
pp. 4219-4225.
ISSN 0974-6846; ESSN: 0974-5645
Abstract
Classification is a well-known supervised learning technique in data mining. It is used to extract meaningful information from large datasets and can be effectively used for predicting unknown classes. In this research, classification is applied to a crime dataset to predict ‘Crime Category’ for different states of the United States of America. The crime dataset used in this research is real in nature, it was collected from socio-economic data from 1990 US Census, law enforcement data from the 1990 US LEMAS survey, and crime data from the 1995 FBI UCR. This paper compares the two different classification algorithms namely, Naïve Bayesian and Decision Tree for predicting ‘Crime Category’ for different states in USA. The results from the experiment showed that, Decision Tree algorithm out performed Naïve Bayesian algorithm and achieved 83.9519% Accuracy in predicting ‘Crime Category’ for different states of USA.
Download File
![[img]](http://psasir.upm.edu.my/30625/1.hassmallThumbnailVersion/An%20experimental%20study%20of%20classification%20algorithms%20for%20crime%20prediction.pdf) 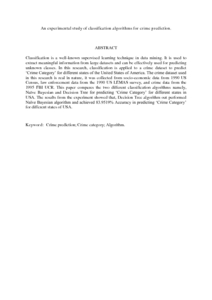 Preview |
|
PDF (Abstract)
An experimental study of classification algorithms for crime prediction.pdf
Download (184kB)
| Preview
|
|
Additional Metadata
Actions (login required)
 |
View Item |